Structured prediction¶
In this example\(\newcommand{\reals}{\mathbf{R}}\)\(\newcommand{\ones}{\mathbf{1}}\), we fit a regression model to structured data, using an LLCP. The training dataset \(\mathcal D\) contains \(N\) input-output pairs \((x, y)\), where \(x \in \reals^{n}_{++}\) is an input and \(y \in \reals^{m}_{++}\) is an outputs. The entries of each output \(y\) are sorted in ascending order, meaning \(y_1 \leq y_2 \leq \cdots \leq y_m\).
Our regression model \(\phi : \reals^{n}_{++} \to \reals^{m}_{++}\) takes as input a vector \(x \in \reals^{n}_{++}\), and solves an LLCP to produce a prediction \(\hat y \in \reals^{m}_{++}\). In particular, the solution of the LLCP is model’s prediction. The model is of the form
Here, the minimization is over \(y \in \reals^{m}_{++}\) and an auxiliary variable \(z \in \reals^{m}_{++}\), \(\phi(x)\) is the optimal value of \(y\), and the parameters are \(c \in \reals^{m}_{++}\) and \(A \in \reals^{m \times n}\). The ratios in the objective are meant elementwise, as is the inequality \(y \leq z\), and \(\ones\) denotes the vector of all ones. Given a vector \(x\), this model finds a sorted vector \(\hat y\) whose entries are close to monomial functions of \(x\) (which are the entries of \(z\)), as measured by the fractional error.
The training loss \(\mathcal{L}(\phi)\) of the model on the training set is the mean squared loss
We emphasize that \(\mathcal{L}(\phi)\) depends on \(c\) and \(A\). In this example, we fit the parameters \(c\) and \(A\) in the LLCP to minimize the training loss \(\mathcal{L}(\phi)\).
Fitting. We fit the parameters by an iterative projected gradient
descent method on \(\mathcal L(\phi)\). In each iteration, we first
compute predictions \(\phi(x)\) for each input in the training set;
this requires solving \(N\) LLCPs. Next, we evaluate the training
loss \(\mathcal L(\phi)\). To update the parameters, we compute the
gradient \(\nabla \mathcal L(\phi)\) of the training loss with
respect to the parameters \(c\) and \(A\). This requires
differentiating through the solution map of the LLCP. We can compute
this gradient efficiently, using the backward
method in CVXPY (or
CVXPY Layers). Finally, we subtract a small multiple of the gradient
from the parameters. Care must be taken to ensure that \(c\) is
strictly positive; this can be done by clamping the entries of \(c\)
at some small threshold slightly above zero. We run this method for a
fixed number of iterations.
This example is described in the paper Differentiating through Log-Log Convex Programs.
Shane Barratt formulated the idea of using an optimization layer to regress on sorted vectors.
Requirements. This example requires PyTorch and CvxpyLayers >= v0.1.3.
from cvxpylayers.torch import CvxpyLayer
import cvxpy as cp
import matplotlib.pyplot as plt
import numpy as np
import torch
torch.set_default_tensor_type(torch.DoubleTensor)
%matplotlib inline
Data generation¶
n = 20
m = 10
# Number of training input-output pairs
N = 100
# Number of validation pairs
N_val = 50
torch.random.manual_seed(243)
np.random.seed(243)
normal = torch.distributions.multivariate_normal.MultivariateNormal(torch.zeros(n), torch.eye(n))
lognormal = lambda batch: torch.exp(normal.sample(torch.tensor([batch])))
A_true = torch.randn((m, n)) / 10
c_true = np.abs(torch.randn(m))
def generate_data(num_points, seed):
torch.random.manual_seed(seed)
np.random.seed(seed)
latent = lognormal(num_points)
noise = lognormal(num_points)
inputs = noise + latent
input_cp = cp.Parameter(pos=True, shape=(n,))
prediction = cp.multiply(c_true.numpy(), cp.gmatmul(A_true.numpy(), input_cp))
y = cp.Variable(pos=True, shape=(m,))
objective_fn = cp.sum(prediction / y + y/prediction)
constraints = []
for i in range(m-1):
constraints += [y[i] <= y[i+1]]
problem = cp.Problem(cp.Minimize(objective_fn), constraints)
outputs = []
for i in range(num_points):
input_cp.value = inputs[i, :].numpy()
problem.solve(cp.SCS, gp=True)
outputs.append(y.value)
return inputs, torch.stack([torch.tensor(t) for t in outputs])
train_inputs, train_outputs = generate_data(N, 243)
plt.plot(train_outputs[0, :].numpy())
[<matplotlib.lines.Line2D at 0x12b367cd0>]
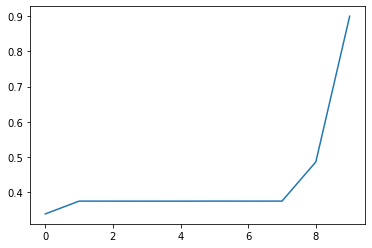
val_inputs, val_outputs = generate_data(N_val, 0)
plt.plot(val_outputs[0, :].numpy())
[<matplotlib.lines.Line2D at 0x12da7e410>]
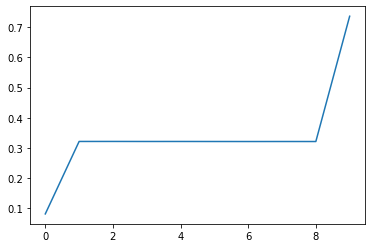
Monomial fit to each component¶
We will initialize the parameters in our LLCP model by fitting monomials to the training data, without enforcing the monotonicity constraint.
log_c = cp.Variable(shape=(m,1))
theta = cp.Variable(shape=(n, m))
inputs_np = train_inputs.numpy()
log_outputs_np = np.log(train_outputs.numpy()).T
log_inputs_np = np.log(inputs_np).T
offsets = cp.hstack([log_c]*N)
cp_preds = theta.T @ log_inputs_np + offsets
objective_fn = (1/N) * cp.sum_squares(cp_preds - log_outputs_np)
lstq_problem = cp.Problem(cp.Minimize(objective_fn))
lstq_problem.is_dcp()
True
lstq_problem.solve(verbose=True)
-----------------------------------------------------------------
OSQP v0.6.0 - Operator Splitting QP Solver
(c) Bartolomeo Stellato, Goran Banjac
University of Oxford - Stanford University 2019
-----------------------------------------------------------------
problem: variables n = 1210, constraints m = 1000
nnz(P) + nnz(A) = 23000
settings: linear system solver = qdldl,
eps_abs = 1.0e-05, eps_rel = 1.0e-05,
eps_prim_inf = 1.0e-04, eps_dual_inf = 1.0e-04,
rho = 1.00e-01 (adaptive),
sigma = 1.00e-06, alpha = 1.60, max_iter = 10000
check_termination: on (interval 25),
scaling: on, scaled_termination: off
warm start: on, polish: on, time_limit: off
iter objective pri res dua res rho time
1 0.0000e+00 3.30e+00 1.22e+04 1.00e-01 3.06e-03s
50 1.0014e-02 1.72e-07 1.64e-07 1.75e-03 7.37e-03s
plsh 1.0014e-02 1.56e-15 1.17e-14 -------- 9.68e-03s
status: solved
solution polish: successful
number of iterations: 50
optimal objective: 0.0100
run time: 9.68e-03s
optimal rho estimate: 8.77e-05
0.010014212812318733
c = torch.exp(torch.tensor(log_c.value)).squeeze()
lstsq_val_preds = []
for i in range(N_val):
inp = val_inputs[i, :].numpy()
pred = cp.multiply(c,cp.gmatmul(theta.T.value, inp))
lstsq_val_preds.append(pred.value)
Fitting¶
A_param = cp.Parameter(shape=(m, n))
c_param = cp.Parameter(pos=True, shape=(m,))
x_slack = cp.Variable(pos=True, shape=(n,))
x_param = cp.Parameter(pos=True, shape=(n,))
y = cp.Variable(pos=True, shape=(m,))
prediction = cp.multiply(c_param, cp.gmatmul(A_param, x_slack))
objective_fn = cp.sum(prediction / y + y / prediction)
constraints = [x_slack == x_param]
for i in range(m-1):
constraints += [y[i] <= y[i+1]]
problem = cp.Problem(cp.Minimize(objective_fn), constraints)
problem.is_dgp(dpp=True)
True
A_param.value = np.random.randn(m, n)
x_param.value = np.abs(np.random.randn(n))
c_param.value = np.abs(np.random.randn(m))
layer = CvxpyLayer(problem, parameters=[A_param, c_param, x_param], variables=[y], gp=True)
torch.random.manual_seed(1)
A_tch = torch.tensor(theta.T.value)
A_tch.requires_grad_(True)
c_tch = torch.tensor(np.squeeze(np.exp(log_c.value)))
c_tch.requires_grad_(True)
train_losses = []
val_losses = []
lam1 = torch.tensor(1e-1)
lam2 = torch.tensor(1e-1)
opt = torch.optim.SGD([A_tch, c_tch], lr=5e-2)
for epoch in range(10):
preds = layer(A_tch, c_tch, train_inputs, solver_args={'acceleration_lookback': 0})[0]
loss = (preds - train_outputs).pow(2).sum(axis=1).mean(axis=0)
with torch.no_grad():
val_preds = layer(A_tch, c_tch, val_inputs, solver_args={'acceleration_lookback': 0})[0]
val_loss = (val_preds - val_outputs).pow(2).sum(axis=1).mean(axis=0)
print('(epoch {0}) train / val ({1:.4f} / {2:.4f}) '.format(epoch, loss, val_loss))
train_losses.append(loss.item())
val_losses.append(val_loss.item())
opt.zero_grad()
loss.backward()
opt.step()
with torch.no_grad():
c_tch = torch.max(c_tch, torch.tensor(1e-8))
(epoch 0) train / val (0.0018 / 0.0014)
(epoch 1) train / val (0.0017 / 0.0014)
(epoch 2) train / val (0.0017 / 0.0014)
(epoch 3) train / val (0.0017 / 0.0014)
(epoch 4) train / val (0.0017 / 0.0014)
(epoch 5) train / val (0.0017 / 0.0014)
(epoch 6) train / val (0.0016 / 0.0014)
(epoch 7) train / val (0.0016 / 0.0014)
(epoch 8) train / val (0.0016 / 0.0014)
(epoch 9) train / val (0.0016 / 0.0014)
with torch.no_grad():
train_preds_tch = layer(A_tch, c_tch, train_inputs)[0]
train_preds = [t.detach().numpy() for t in train_preds_tch]
with torch.no_grad():
val_preds_tch = layer(A_tch, c_tch, val_inputs)[0]
val_preds = [t.detach().numpy() for t in val_preds_tch]
fig = plt.figure()
i = 0
plt.plot(val_preds[i], label='LLCP', color='teal')
plt.plot(lstsq_val_preds[i], label='least squares', linestyle='--', color='gray')
plt.plot(val_outputs[i], label='true', linestyle='-.', color='orange')
w, h = 8, 3.5
plt.xlabel(r'$i$')
plt.ylabel(r'$y_i$')
plt.legend()
plt.show()
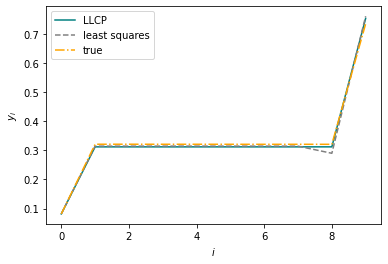