Fault detection¶
We’ll consider a problem of identifying faults that have occurred in a system based on sensor measurements of system performance.
Topic references¶
Problem statement¶
Each of \(n\) possible faults occurs independently with probability \(p\). The vector \(x \in \lbrace 0,1 \rbrace^{n}\) encodes the fault occurrences, with \(x_i = 1\) indicating that fault \(i\) has occurred. System performance is measured by \(m\) sensors. The sensor output is
where \(A \in \mathbf{R}^{m \times n}\) is the sensing matrix with column \(a_i\) being the fault signature of fault \(i\), and \(v \in \mathbf{R}^m\) is a noise vector where \(v_j\) is Gaussian with mean 0 and variance \(\sigma^2\).
The objective is to guess \(x\) (which faults have occurred) given \(y\) (sensor measurements).
We are interested in the setting where \(n > m\), that is, when we have more possible faults than measurements. In this setting, we can expect a good recovery when the vector \(x\) is sparse. This is the subject of compressed sensing.
Solution approach¶
To identify the faults, one reasonable approach is to choose \(x \in \lbrace 0,1 \rbrace^{n}\) to minimize the negative log-likelihood function
However, this problem is nonconvex and NP-hard, due to the constraint that \(x\) must be Boolean.
To make this problem tractable, we can relax the Boolean constraints and instead constrain \(x_i \in [0,1]\).
The optimization problem
is convex. We’ll refer to the solution of the convex problem as the relaxed ML estimate.
By taking the relaxed ML estimate of \(x\) and rounding the entries to the nearest of 0 or 1, we recover a Boolean estimate of the fault occurrences.
Example¶
We’ll generate an example with \(n = 2000\) possible faults, \(m = 200\) measurements, and fault probability \(p = 0.01\). We’ll choose \(\sigma^2\) so that the signal-to-noise ratio is 5. That is,
import numpy as np
import matplotlib.pyplot as plt
np.random.seed(1)
n = 2000
m = 200
p = 0.01
snr = 5
sigma = np.sqrt(p*n/(snr**2))
A = np.random.randn(m,n)
x_true = (np.random.rand(n) <= p).astype(int)
v = sigma*np.random.randn(m)
y = A.dot(x_true) + v
Below, we show \(x\), \(Ax\) and the noise \(v\).
plt.plot(range(n),x_true)
[<matplotlib.lines.Line2D at 0x11ae42518>]
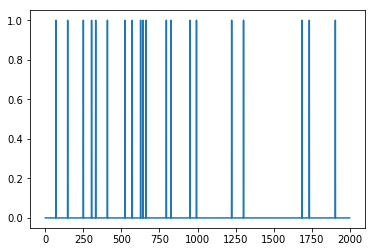
plt.plot(range(m), A.dot(x_true),range(m),v)
plt.legend(('Ax','v'))
<matplotlib.legend.Legend at 0x11aee9630>
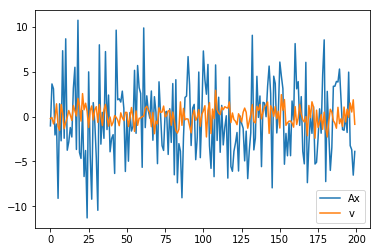
Recovery¶
We solve the relaxed maximum likelihood problem with CVXPY and then round the result to get a Boolean solution.
%%time
import cvxpy as cp
x = cp.Variable(shape=n)
tau = 2*cp.log(1/p - 1)*sigma**2
obj = cp.Minimize(cp.sum_squares(A*x - y) + tau*cp.sum(x))
const = [0 <= x, x <= 1]
cp.Problem(obj,const).solve(verbose=True)
print("final objective value: {}".format(obj.value))
# relaxed ML estimate
x_rml = np.array(x.value).flatten()
# rounded solution
x_rnd = (x_rml >= .5).astype(int)
ECOS 2.0.4 - (C) embotech GmbH, Zurich Switzerland, 2012-15. Web: www.embotech.com/ECOS
It pcost dcost gap pres dres k/t mu step sigma IR | BT
0 +7.343e+03 -3.862e+03 +5e+04 5e-01 5e-04 1e+00 1e+01 --- --- 1 1 - | - -
1 +4.814e+02 -9.580e+02 +8e+03 1e-01 6e-05 2e-01 2e+00 0.8500 1e-02 1 2 2 | 0 0
2 -2.079e+02 -1.428e+03 +6e+03 1e-01 4e-05 8e-01 2e+00 0.7544 7e-01 2 2 2 | 0 0
3 -1.321e+02 -1.030e+03 +5e+03 8e-02 3e-05 7e-01 1e+00 0.3122 2e-01 2 2 2 | 0 0
4 -2.074e+02 -8.580e+02 +4e+03 6e-02 2e-05 6e-01 9e-01 0.7839 7e-01 2 2 2 | 0 0
5 -1.121e+02 -6.072e+02 +3e+03 5e-02 1e-05 5e-01 7e-01 0.3859 4e-01 2 3 3 | 0 0
6 -4.898e+01 -4.060e+02 +2e+03 3e-02 8e-06 3e-01 5e-01 0.5780 5e-01 2 2 2 | 0 0
7 +7.778e+01 -5.711e+01 +8e+02 1e-02 3e-06 1e-01 2e-01 0.9890 4e-01 2 3 2 | 0 0
8 +1.307e+02 +6.143e+01 +4e+02 6e-03 1e-06 6e-02 1e-01 0.5528 1e-01 3 3 3 | 0 0
9 +1.607e+02 +1.286e+02 +2e+02 3e-03 4e-07 3e-02 5e-02 0.8303 3e-01 3 3 3 | 0 0
10 +1.741e+02 +1.557e+02 +1e+02 2e-03 2e-07 2e-02 3e-02 0.6242 3e-01 3 3 3 | 0 0
11 +1.834e+02 +1.749e+02 +5e+01 8e-04 9e-08 8e-03 1e-02 0.8043 3e-01 3 3 3 | 0 0
12 +1.888e+02 +1.861e+02 +2e+01 3e-04 3e-08 2e-03 4e-03 0.9175 3e-01 3 3 2 | 0 0
13 +1.909e+02 +1.902e+02 +4e+00 7e-05 7e-09 6e-04 1e-03 0.8198 1e-01 3 3 3 | 0 0
14 +1.914e+02 +1.912e+02 +1e+00 2e-05 2e-09 2e-04 3e-04 0.8581 2e-01 3 2 3 | 0 0
15 +1.916e+02 +1.916e+02 +1e-01 2e-06 3e-10 2e-05 4e-05 0.9004 3e-02 3 3 3 | 0 0
16 +1.916e+02 +1.916e+02 +4e-02 7e-07 8e-11 7e-06 1e-05 0.8174 1e-01 3 3 3 | 0 0
17 +1.916e+02 +1.916e+02 +8e-03 1e-07 1e-11 1e-06 2e-06 0.8917 9e-02 3 2 2 | 0 0
18 +1.916e+02 +1.916e+02 +2e-03 4e-08 4e-12 4e-07 5e-07 0.8588 2e-01 3 3 3 | 0 0
19 +1.916e+02 +1.916e+02 +2e-04 3e-09 3e-13 3e-08 5e-08 0.9309 2e-02 3 2 2 | 0 0
20 +1.916e+02 +1.916e+02 +2e-05 4e-10 4e-14 4e-09 6e-09 0.8768 1e-02 4 2 2 | 0 0
21 +1.916e+02 +1.916e+02 +4e-06 6e-11 6e-15 6e-10 9e-10 0.9089 6e-02 4 2 2 | 0 0
22 +1.916e+02 +1.916e+02 +1e-06 2e-11 2e-15 2e-10 2e-10 0.8362 1e-01 2 1 1 | 0 0
OPTIMAL (within feastol=1.8e-11, reltol=5.1e-09, abstol=9.8e-07).
Runtime: 6.538894 seconds.
final objective value: 191.6347201927456
CPU times: user 6.51 s, sys: 291 ms, total: 6.8 s
Wall time: 7.5 s
Evaluation¶
We define a function for computing the estimation errors, and a function for plotting \(x\), the relaxed ML estimate, and the rounded solutions.
import matplotlib
def errors(x_true, x, threshold=.5):
'''Return estimation errors.
Return the true number of faults, the number of false positives, and the number of false negatives.
'''
n = len(x_true)
k = sum(x_true)
false_pos = sum(np.logical_and(x_true < threshold, x >= threshold))
false_neg = sum(np.logical_and(x_true >= threshold, x < threshold))
return (k, false_pos, false_neg)
def plotXs(x_true, x_rml, x_rnd, filename=None):
'''Plot true, relaxed ML, and rounded solutions.'''
matplotlib.rcParams.update({'font.size': 14})
xs = [x_true, x_rml, x_rnd]
titles = ['x_true', 'x_rml', 'x_rnd']
n = len(x_true)
k = sum(x_true)
fig, ax = plt.subplots(1, 3, sharex=True, sharey=True, figsize=(12, 3))
for i,x in enumerate(xs):
ax[i].plot(range(n), x)
ax[i].set_title(titles[i])
ax[i].set_ylim([0,1])
if filename:
fig.savefig(filename, bbox_inches='tight')
return errors(x_true, x_rml,.5)
We see that out of 20 actual faults, the rounded solution gives perfect recovery with 0 false negatives and 0 false positives.
plotXs(x_true, x_rml, x_rnd, 'fault.pdf')
(20, 0, 0)
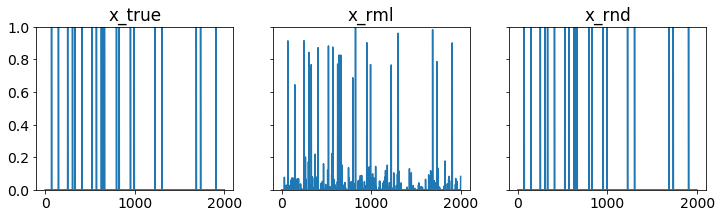